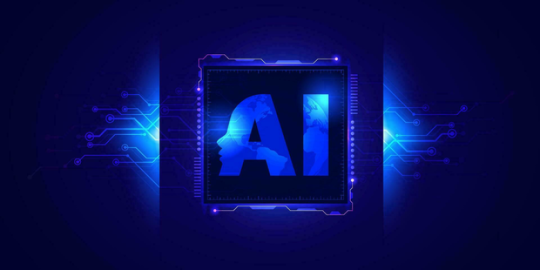
The journey towards widespread AI adoption is fraught with challenges. While AI's potential to enhance consumer experiences and streamline business operations through personalization, autonomy, and decentralized reasoning is clear, the technology carries inherent risks. AI can produce misleading conclusions, spread misinformation, and sometimes reinforce existing biases. This darker side of AI can lead to significant financial, legal, and reputational damage for businesses.
Lost in the Digital Mirage
AI is susceptible to hallucinations—instances where models draw conclusions that, while coherent, are disconnected from reality or the input's context. Similar to human hallucinations, AI can experience digital mirages, blurring the lines between what's real and what's not. For example, an earlier version of ChatGPT once claimed that the Golden Gate Bridge was relocated to Egypt in 2016. Such imaginative and unexpected outputs highlight that AI's conclusions can be error-prone and misleading.
Not all AI hallucinations are harmless. Businesses relying on flawed AI outputs can face costly errors. In critical sectors like healthcare, this can be a matter of life or death. Imagine an AI system designed to detect heart conditions that hallucinates a heartbeat irregularity, leading to incorrect diagnoses, unnecessary medical interventions, and potential harm to patient safety.
Bias in AI Models
AI models trained on biased datasets can lead to significant issues, as evidenced by Amazon's AI-driven recruitment tool. This tool unintentionally discriminated against female candidates because it was based on data that mirrored historical and societal biases. Rather than selecting the most qualified applicants, the tool reinforced existing inequalities. This case highlights how AI can perpetuate and magnify real-world biases, potentially leading to worse outcomes in the future. The absence of thorough AI regulations can make these problems more severe, leaving companies without clear directives on how to prevent them.
Consequently, businesses can face lawsuits for negligence or discrimination, resulting in fines. Financial losses may stem from erroneous decisions and missed opportunities, while an untrustworthy AI model can drive customers away. These issues could lead organizations to deem AI too risky, abandoning planned projects. In scenarios where AI would have increased productivity, abandoning these projects might leave staff with additional workloads.
RAG to the Rescue
Despite these challenges, AI offers significant opportunities for businesses. By adopting the right tools, models, and best practices, companies can effectively address potential issues and fully leverage AI's capabilities. Retrieval-augmented generation (RAG) models are instrumental in addressing problems related to bias, fairness, and hallucinations. RAG models integrate retrieval mechanisms that enable access to extensive, diverse, and relevant data, helping to reduce the impact of biases found in smaller or more restricted datasets.
RAG models are exceptional at producing responses based on accurate information, effectively reducing AI hallucinations by referencing context-specific data from trustworthy sources. These models can be tailored for particular tasks or scenarios, offering users information that is uniquely relevant to their needs. This capability is particularly advantageous for businesses that do not have the time or resources to retrain AI models with specialized datasets.
In the recruitment example mentioned earlier, RAG can help inform the model with updated hiring guidelines, HR policies, and equal employment opportunity laws, ensuring a balanced approach for fair and equal treatment of all candidates.
Practical Applications of RAG
Beyond retrieving relevant information, RAG models generate natural, conversational responses, enhancing user-friendliness for customer-facing applications. RAG models should be a key component of a modern data strategy. To maximize their effectiveness, RAG models require access to real-time data to ensure information is current and accurate. They should also be paired with an operational data store that holds information in high-dimensional mathematical vectors. This allows models to convert user queries into numerical vectors, enabling AI to respond to relevant text inquiries even if they don't precisely match the original terms or phrases.
By using real-time data and vector-based databases, AI model outputs remain up-to-date, reducing the risk of outdated information leading to hallucinations.
Heading Towards a Brighter Future
As we move towards widespread AI adoption, organizations must recognize the consequences of AI gone wrong. A comprehensive approach to AI is essential, paving the way for ethical, fair, and secure systems that enhance productivity and personalization without spreading misinformation. By prioritizing responsible AI practices, such as employing RAG models, businesses can ensure AI remains a positive force in society, rather than something to be feared.